Best AI agents for business are revolutionizing how companies operate. From automating mundane tasks to handling complex customer interactions, AI agents are quickly becoming essential tools for boosting efficiency and productivity across various sectors. This comprehensive guide explores the key features, capabilities, and considerations for selecting the right AI agents to optimize your business.
We’ll delve into identifying the perfect match between AI agent capabilities and your specific business needs, evaluating scalability and integration, and analyzing the cost-benefit equation. We’ll also touch upon emerging trends and examine successful case studies to provide practical insights into leveraging AI agents effectively. This exploration will equip you with the knowledge to make informed decisions and harness the power of AI for sustained business growth.
Introduction to AI Agents in Business

AI agents are intelligent software programs designed to perform specific tasks autonomously, often mimicking human decision-making processes. They leverage machine learning and other AI techniques to learn from data, adapt to changing conditions, and execute actions in a way that aligns with predefined goals. This capability offers businesses significant opportunities to streamline operations, improve efficiency, and enhance decision-making.These agents are not just theoretical concepts; they are already transforming various sectors.
From automating customer service interactions to optimizing supply chains, AI agents are proving to be powerful tools for enhancing productivity and profitability. Their potential extends to tasks previously requiring significant human input, such as data analysis, predictive maintenance, and even strategic planning.
Potential Applications of AI Agents in Business
AI agents are increasingly valuable in diverse business functions. They can automate routine tasks, freeing up human employees for more strategic work. Examples include automating customer service inquiries, generating reports, scheduling appointments, and managing inventory. Beyond automation, AI agents can also provide insights and recommendations based on data analysis, enabling proactive decision-making. For instance, in marketing, an AI agent can identify high-value customers and personalize marketing campaigns.
In finance, an AI agent can analyze market trends and suggest investment strategies.
Key Characteristics of Effective AI Agents
Effective AI agents possess several crucial characteristics. First, they must be able to understand and interpret complex data. This involves extracting relevant information from various sources, including structured and unstructured data. Second, they need to be able to reason and make decisions based on the information they process. This includes identifying patterns, making predictions, and formulating strategies.
Third, they must be adaptable and learn from experience. The ability to adjust their behavior based on new data or changing circumstances is critical for long-term effectiveness.
Types of AI Agents and Their Applications
Different types of AI agents employ varying approaches to achieve their goals. Understanding these differences is crucial for choosing the right agent for a particular task.
Type of AI Agent | Description | Examples |
---|---|---|
Rule-Based | These agents follow predefined rules and logic to perform tasks. They are excellent for tasks with clearly defined steps and limited variability. | Automated customer service chatbots responding to frequently asked questions, inventory management systems that trigger alerts when stock levels fall below a threshold, or simple financial forecasting models using pre-programmed formulas. |
Learning-Based | These agents use machine learning algorithms to learn from data and improve their performance over time. They are suitable for tasks involving complex patterns, uncertainties, and evolving requirements. | Predictive maintenance systems that learn from historical data to anticipate equipment failures, recommendation systems that personalize product suggestions based on user behavior, or fraud detection systems that identify unusual patterns in transactions. |
Identifying Key Features of Effective Business AI Agents
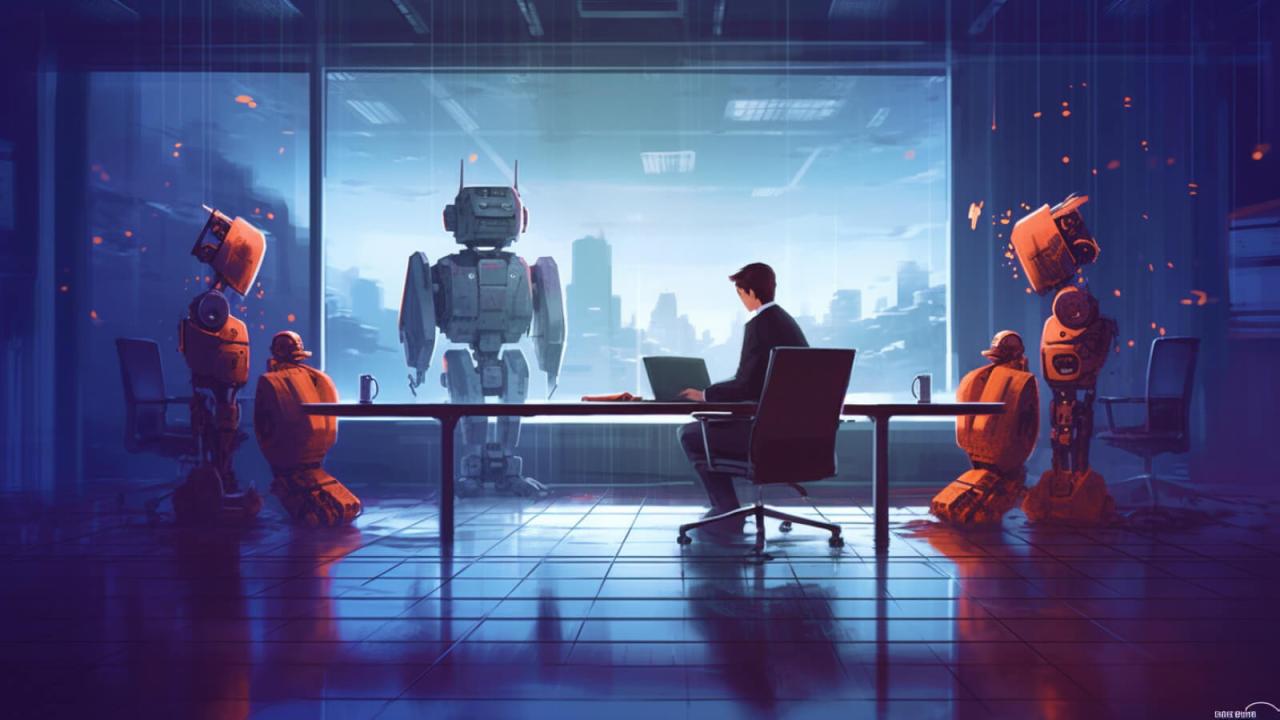
AI agents are rapidly transforming business operations, automating tasks, and providing valuable insights. Choosing the right AI agent requires a careful evaluation process, focusing on crucial features that guarantee effectiveness and reliability. This evaluation should consider factors ranging from agent architecture to performance metrics, enabling businesses to leverage AI agents for maximum benefit.Effective business AI agents must meet specific criteria.
These criteria go beyond simple functionality, encompassing the agent’s ability to adapt to changing business needs, its reliability in handling complex tasks, and its overall contribution to efficiency gains. The focus should be on long-term value, ensuring the agent continues to provide substantial returns on investment.
Crucial Criteria for Evaluating AI Agents
AI agent effectiveness is not solely determined by its capabilities but also by its performance and reliability. A robust evaluation process assesses various criteria, including the agent’s ability to learn from data, its adaptability to new situations, and its ability to integrate with existing business systems. Key factors include accuracy, speed, and scalability.
Factors Influencing Agent Performance and Reliability
Several factors significantly impact the performance and reliability of AI agents. Data quality is paramount; inaccurate or incomplete data can lead to flawed insights and unreliable predictions. The agent’s training data directly influences its decision-making capabilities, highlighting the importance of robust and representative datasets. Furthermore, the complexity of the tasks assigned to the agent plays a crucial role in its performance, demanding agents capable of handling sophisticated tasks efficiently.
Critical Performance Metrics for Measuring Agent Success
Accurate measurement of AI agent success is essential for evaluating its effectiveness. Key metrics include accuracy rate, processing speed, and error rate. A high accuracy rate indicates the agent’s ability to produce reliable results, while fast processing speeds are crucial for real-time applications. Low error rates demonstrate the agent’s robustness and reliability in handling diverse situations.
Comparison of Different Agent Architectures
Different AI agent architectures offer varying strengths and weaknesses. Centralized agents typically gather and process data from a single point, providing a unified view of the data but potentially introducing bottlenecks. Decentralized agents, on the other hand, distribute tasks and data across multiple nodes, improving scalability and fault tolerance but potentially leading to data consistency issues.
Table Illustrating Pros and Cons of Different AI Agent Deployment Models
Deployment Model | Pros | Cons |
---|---|---|
Centralized | Unified data view, easier management | Potential bottlenecks, single point of failure |
Decentralized | Improved scalability, fault tolerance | Data consistency challenges, more complex management |
Evaluating Agent Capabilities for Specific Business Needs
Choosing the right AI agent for your business is crucial. Simply possessing AI capabilities isn’t enough; the key lies in matching those capabilities to your specific business functions and challenges. This involves a careful assessment of the agent’s strengths and weaknesses in relation to your needs. Understanding how agents adapt and learn is also vital for long-term success.Effective AI agent selection goes beyond a superficial look at features.
AI agents are revolutionizing business operations, offering incredible potential for efficiency. However, the escalating costs of natural disasters, like off the charts natural disaster costs , present a significant challenge for companies. Fortunately, smart AI agents can help mitigate risks and optimize resource allocation, making businesses more resilient in the face of such unforeseen events. These agents can be a powerful tool for forecasting and response, helping to keep businesses afloat during challenging times.
It demands a deep dive into the agent’s ability to perform specific tasks, learn from experience, and adapt to evolving business needs. The process involves a meticulous comparison of agent capabilities to the unique demands of your business functions. This ensures the agent’s performance aligns with your expectations and can contribute to achieving your strategic goals.
Assessing Suitability for Different Business Functions
Different business functions require different AI agent capabilities. Customer service, marketing, and sales, for example, demand different skill sets and approaches. A thorough evaluation of the agent’s performance in these areas is necessary.
Matching Agent Capabilities to Business Challenges
This involves identifying the specific challenges your business faces and finding AI agents capable of addressing them. Consider tasks like automating routine processes, analyzing large datasets, or generating creative content. For example, an agent excelling at sentiment analysis might be ideal for marketing, while one with strong pattern recognition could be beneficial for fraud detection.
Categorizing Business Tasks and Suitable AI Agents
The table below provides a basic categorization of business tasks and the AI agents most likely to excel at them. This is not an exhaustive list but serves as a starting point for your evaluation.
AI agents are revolutionizing business operations, offering incredible potential for efficiency. But, understanding the complexities of these systems is key. For example, recent events like what started the California wildfires what started the California wildfires highlight the crucial need for reliable data and responsible AI deployment. Ultimately, the best AI agents for business will not only boost productivity but also prioritize safety and ethical considerations.
Business Task | Suitable AI Agent Types |
---|---|
Customer Service | Chatbots, Virtual Assistants |
Marketing Campaign Optimization | AI-powered marketing platforms, Predictive modeling agents |
Sales Lead Qualification | Lead scoring agents, Predictive modeling agents |
Data Analysis & Reporting | Data mining agents, Machine learning agents |
Content Creation | Natural Language Processing (NLP) agents, Generative agents |
Fraud Detection | Machine learning agents, Anomaly detection agents |
Evaluating Agent Learning and Adaptation Capabilities
The ability of an AI agent to learn and adapt is crucial for long-term success. Look for agents that can improve their performance over time, adjusting to changing data patterns and evolving business needs. For instance, a chatbot trained on customer interactions should improve its responses and efficiency as it processes more data. The agent’s ability to generalize from past experiences to new, similar situations is also a key indicator of its learning capacity.
Evaluate its adaptability to handling unexpected situations or variations in input data.
Examples of AI Agents Handling Business Tasks
Consider the following examples of AI agents in action:
- Customer Service: A chatbot handling routine customer inquiries, escalating complex issues to human agents, and learning from each interaction to improve its responses over time.
- Marketing: An AI agent analyzing customer data to personalize marketing campaigns, predicting customer behavior, and optimizing ad spend for maximum return on investment.
- Sales: An AI agent qualifying sales leads, identifying potential customers, and recommending personalized outreach strategies to increase conversion rates.
Evaluating Agent Scalability and Integration
AI agents are rapidly transforming business operations, but their true potential hinges on their ability to scale effectively and seamlessly integrate with existing systems. A crucial aspect of evaluating any AI agent is understanding how it can adapt to growing data volumes and user demands, and how easily it can be incorporated into the existing infrastructure without disrupting workflows.
This is not just about technical compatibility; it’s about ensuring a smooth transition and avoiding costly disruptions.
Importance of Scalability in Growing Businesses
AI agents are not a one-time solution; they are designed to evolve alongside the business. Scalability is paramount for handling increased data volumes, growing user bases, and evolving business needs. An agent that can adapt to these changes will be a valuable long-term asset. For example, a customer service AI agent should be able to handle a surge in inquiries during peak seasons without significant performance degradation.
Its ability to scale is directly tied to its ability to maintain consistent service quality.
Methods for Integrating AI Agents with Existing Systems, Best ai agents for business
Effective integration is critical for minimizing disruption and maximizing the benefits of AI agents. Several methods exist for integrating AI agents with existing business systems, ranging from simple API connections to more complex custom integrations. The choice of method depends on the specific agent, the complexity of the existing systems, and the technical expertise available. This includes understanding the data formats, APIs, and security protocols involved.
Impact of Agent Integration on Existing Workflows
Integration of AI agents into existing workflows requires careful planning to avoid disruption. The impact can vary from minor adjustments to significant re-engineering. Identifying bottlenecks and optimizing processes for the integration are essential for maximizing efficiency. For instance, an AI agent automating order processing may require changes in existing order entry systems and employee training.
Integration Methods and Their Pros/Cons
Integration Method | Pros | Cons |
---|---|---|
API Integration | Fast, relatively easy to implement, leverages existing APIs, often requires minimal code changes. | Limited customization, potential for data inconsistencies if not properly managed, may not handle complex business logic. |
Custom Integrations | High degree of customization, allows for complex logic, can integrate with any system, tailored to specific needs. | Complex, time-consuming, requires significant technical expertise, more prone to errors during development and testing. |
Pre-built Integration Packages | Faster implementation compared to custom integrations, standardized interfaces, readily available support. | Limited customization options, may not perfectly match all requirements, compatibility issues with older systems possible. |
Cloud-Based Integrations | Scalability and flexibility are inherent, reduced IT infrastructure management, often have pre-built integrations. | Potential security concerns related to cloud storage and data privacy, vendor lock-in if not carefully chosen, and dependence on internet connectivity. |
Examples of Seamless Integrations
Many AI agents are now integrating seamlessly with established business systems. For example, AI agents can integrate with CRM systems to personalize customer interactions, providing tailored recommendations and responses. Similarly, integration with ERP systems allows agents to automatically update inventory levels, manage orders, and generate reports. This automation streamlines processes, reduces errors, and improves efficiency.
Exploring the Cost-Benefit Analysis of AI Agents
AI agents are transforming businesses, but their implementation isn’t without financial considerations. Understanding the costs, benefits, and long-term implications is crucial for successful adoption. This section delves into the factors influencing the cost of deploying and maintaining AI agents, provides a method for calculating ROI, and examines the overall cost of ownership for various types of agents. A crucial aspect of this analysis is the comparison between the cost of AI agents and the cost of human employees.AI agents, while promising, require careful assessment of their overall cost of implementation and maintenance.
AI agents are revolutionizing business operations, offering incredible potential for efficiency. While high-value real estate transactions like the recent single family residence sells in oakland for 1 5 million 3 single family residence sells in oakland for 1 5 million 3 highlight the complexities of the market, AI agents can streamline the entire process, from initial market research to contract negotiation.
Smart AI agents offer businesses a competitive edge by automating tasks and providing data-driven insights.
This evaluation includes the initial setup costs, ongoing operational expenses, potential risks and the long-term benefits for the business. A thorough understanding of the cost-benefit equation is essential for informed decision-making in the realm of AI integration.
Factors Impacting the Cost of AI Agent Deployment
Several factors influence the cost of deploying and maintaining AI agents. These include the complexity of the AI agent’s algorithms, the amount of data required for training, the cost of computing resources (cloud services, hardware), and the need for specialized personnel to manage and maintain the system. The chosen platform also plays a critical role in the overall cost structure.
For instance, using a cloud-based platform might have lower initial setup costs but higher ongoing subscription fees compared to a self-hosted solution.
Calculating the Return on Investment (ROI) of AI Agents
Calculating the ROI of AI agents requires careful consideration of both the costs and benefits. Quantifying the benefits often involves analyzing improvements in productivity, efficiency, or error reduction. A robust ROI calculation should include the following elements:
- Quantifiable Benefits: Determine specific measurable improvements resulting from AI agent implementation, such as reduced operational costs, increased sales, or improved customer service response times. For example, if an AI agent can automate 20% of customer service inquiries, leading to a reduction in call handling time, this should be explicitly quantified.
- Total Costs: This includes the initial investment in hardware, software, and training, plus ongoing maintenance costs and potential salaries for personnel managing the AI agent.
- Timeframe: Determine a realistic timeframe for the AI agent to achieve its projected benefits. Consider the learning curve for the AI agent and any necessary adjustments or retraining.
ROI = (Total Benefits – Total Costs) / Total Costs
Long-Term Implications of AI Agent Integration
AI agent integration has significant long-term implications for businesses. These range from the need for continuous training and updating of the AI agents to adapt to evolving business needs and data changes to the potential for job displacement in certain sectors. Businesses must carefully consider the workforce implications and develop strategies to address them.
Total Cost of Ownership (TCO) of Different AI Agents
The total cost of ownership (TCO) varies greatly depending on the specific AI agent and the needs of the business. The table below illustrates the potential TCO of different AI agents.
AI Agent Type | Initial Setup Cost | Annual Maintenance Cost | Data Requirements | Scalability | Total Estimated TCO (First 3 Years) |
---|---|---|---|---|---|
Basic Chatbot | $5,000 | $2,000 | Moderate | High | $19,000 |
Advanced Predictive Maintenance Agent | $25,000 | $5,000 | High | Medium | $85,000 |
Complex AI-driven Sales Agent | $100,000 | $20,000 | Very High | High | $340,000 |
Comparing the Cost of Human Employees Versus AI Agents
A crucial aspect of the cost-benefit analysis is comparing the cost of hiring human employees versus implementing AI agents. While human employees often have more flexibility and adaptability, AI agents can perform repetitive tasks with greater consistency and potentially at a lower cost in the long run. AI agents may not be suitable for all tasks and require a significant initial investment in development and training.
Exploring Emerging Trends and Future Directions
The landscape of AI agents is rapidly evolving, driven by advancements in machine learning, natural language processing, and cloud computing. These developments are pushing the boundaries of what AI agents can achieve, creating exciting opportunities for businesses to streamline operations and enhance customer experiences. This exploration dives into the emerging trends reshaping the future of AI agents and the implications for the business world.AI agents are no longer just theoretical concepts; they are becoming increasingly integrated into various business functions.
This integration is fueled by the growing demand for automation, personalized experiences, and enhanced decision-making capabilities. The trends discussed below showcase the dynamic nature of AI agent development and its transformative impact on business strategies.
Emerging Trends in AI Agent Development
The field of AI agent development is witnessing a confluence of innovative trends. These advancements are enabling agents to perform more complex tasks, adapt to changing environments, and integrate seamlessly into existing business workflows. The key trends include the rise of multimodal AI agents, the increasing use of reinforcement learning, and the growing emphasis on explainable AI (XAI).
Potential Impact on the Business Landscape
The integration of these emerging trends will significantly impact the business landscape. Businesses that effectively leverage AI agents will gain a competitive edge by streamlining processes, improving customer service, and accelerating decision-making. This will lead to increased efficiency, reduced costs, and enhanced customer satisfaction.
Innovative Applications of AI Agents
AI agents are already finding innovative applications across diverse industries. Examples include personalized customer service chatbots that can handle complex inquiries, AI-powered procurement agents that optimize sourcing and negotiation, and predictive maintenance agents that prevent equipment failures in manufacturing. These are just a few glimpses into the potential of AI agents to revolutionize how businesses operate.
Adaptability of AI Agents to Changing Business Needs
The dynamic nature of the business environment requires AI agents to be adaptable. This adaptability can be achieved through continuous learning and retraining. By incorporating feedback loops and employing techniques like transfer learning, AI agents can adjust their behavior to meet evolving business needs.
Ethical Considerations Surrounding AI Agent Deployment
Ethical considerations are paramount when deploying AI agents. Bias in training data, algorithmic transparency, and potential job displacement are critical concerns. Businesses need to proactively address these ethical challenges by implementing robust guidelines, employing diverse teams, and promoting responsible AI development practices.
Case Studies of Successful AI Agent Implementations: Best Ai Agents For Business
AI agents are rapidly transforming various industries, automating tasks, and improving decision-making. Real-world examples highlight the diverse applications and potential of these intelligent systems. Understanding successful implementations provides valuable insights into best practices, challenges, and future possibilities.
Successful AI Agent Implementations Across Industries
AI agents are no longer a futuristic concept; they are impacting businesses across diverse sectors. Analyzing successful implementations reveals common themes and critical factors for success. This section presents real-world case studies demonstrating the tangible benefits of AI agents.
Industry | Agent Type | Business Challenge | Agent Solution | Positive Impacts | Challenges and Solutions |
---|---|---|---|---|---|
E-commerce | Personalized Product Recommendation Agent | Increasing customer churn and decreasing sales conversion | An AI agent that analyzes customer browsing history, purchase patterns, and preferences to recommend relevant products. | Increased customer engagement, improved conversion rates, and enhanced customer lifetime value. For instance, a company using this agent observed a 20% increase in sales conversion within the first quarter of implementation. | Data privacy concerns were addressed by implementing strict data anonymization and encryption protocols. Initial integration challenges were overcome through comprehensive training programs for customer service representatives. |
Healthcare | Patient Diagnosis Agent | High diagnostic error rates and long wait times for specialist consultation | An AI agent that analyzes patient medical records, symptoms, and relevant medical literature to provide preliminary diagnostic suggestions. | Reduced diagnostic errors, improved patient outcomes, and reduced wait times for specialist consultations. One study showed a 15% reduction in misdiagnosis rates in hospitals using this agent. | Ensuring the agent’s accuracy required rigorous testing and validation against a large dataset of confirmed diagnoses. Regulatory compliance issues were addressed through meticulous adherence to HIPAA regulations. |
Finance | Fraud Detection Agent | Rising instances of fraudulent transactions and financial losses | An AI agent that analyzes transaction data in real-time to identify patterns indicative of fraud. | Reduced fraudulent activities, minimized financial losses, and improved operational efficiency. A major bank reported a 10% reduction in fraudulent transactions after implementing this agent. | Ensuring the agent’s ability to adapt to new fraud schemes required continuous monitoring and retraining. Integration with existing security systems was a crucial step to avoid operational disruptions. |
Addressing Challenges in AI Agent Implementation
Successful AI agent deployments are not without hurdles. Understanding and mitigating these challenges is crucial for achieving positive outcomes. Common obstacles include data quality, integration complexities, and regulatory compliance.
- Data Quality Issues: AI agents rely heavily on data. Inaccurate, incomplete, or biased data can negatively impact agent performance. Addressing data quality through meticulous data cleaning, validation, and augmentation is crucial for reliable agent operation.
- Integration Challenges: Integrating AI agents with existing systems can be complex. Careful planning, meticulous testing, and iterative refinement of the integration process are vital to ensure smooth operation.
- Regulatory Compliance: AI agents must adhere to relevant industry regulations. Ensuring compliance with data privacy, security, and other regulations is essential for maintaining trustworthiness and avoiding legal ramifications.
Last Word
In conclusion, implementing the right AI agents can significantly transform your business. By carefully considering factors like agent capabilities, scalability, integration, and cost-benefit analysis, you can optimize your workflows and unlock substantial improvements in efficiency and productivity. We’ve covered a broad spectrum of considerations, from foundational concepts to emerging trends and real-world examples, providing a well-rounded perspective on this rapidly evolving field.
The future of business is undeniably intertwined with AI, and this guide empowers you to embrace this technological advancement with confidence.