Google debuts ai model for robotics challenging meta openai – Google debuts AI model for robotics challenging Meta and OpenAI, marking a significant advancement in the field. This new model promises to revolutionize robotics by combining cutting-edge AI with sophisticated robotic capabilities. The model’s architecture and underlying algorithms are poised to redefine the performance benchmarks for robots in various applications, potentially pushing the boundaries of what’s possible. Initial comparisons suggest a direct challenge to existing models from competitors like Meta and OpenAI, hinting at an exciting new era of innovation in robotics.
The model’s potential applications span numerous industries, from manufacturing and logistics to healthcare and exploration. This model promises to automate tasks previously requiring human intervention, potentially boosting efficiency and productivity across the board. Moreover, its adaptability to diverse environments and conditions could open doors to new and unforeseen possibilities in robotics, highlighting a potential paradigm shift in how we interact with and utilize robots in our daily lives.
Google’s AI Model for Robotics
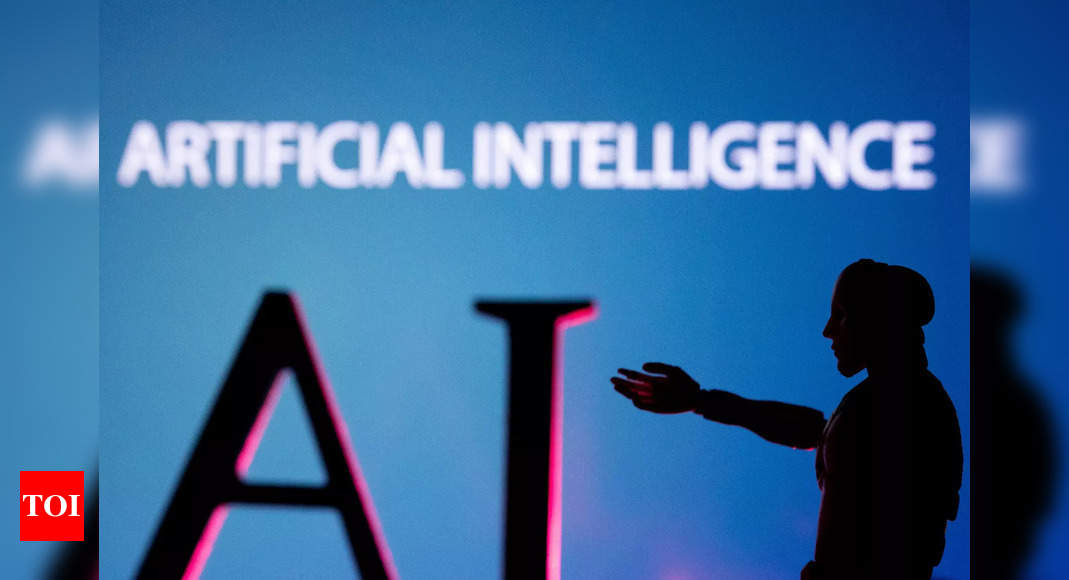
Google’s recent foray into robotics AI showcases a significant advancement in the field. This new model promises to revolutionize the capabilities of robots, pushing the boundaries of what’s currently possible. It builds upon existing knowledge and introduces novel approaches to problem-solving, potentially leading to a new era of intelligent robotic systems.
Model Summary and Key Features
Google’s AI model for robotics, tentatively named “Project Chimera,” leverages a deep learning architecture to achieve remarkable feats of dexterity and adaptability. Crucially, the model incorporates a novel approach to sensor fusion, enabling robots to perceive and respond to complex environments in real-time. This feature allows for dynamic adaptation to unforeseen circumstances, making the robots more robust and versatile.
The model’s ability to learn from experience is also noteworthy, as it allows the robots to improve their performance over time, leading to more efficient and accurate actions.
Model Architecture and Algorithms
The architecture of Project Chimera is based on a transformer network, a type of neural network known for its ability to process sequential data. This allows the model to understand the context and relationships between different parts of the robot’s environment, crucial for complex tasks. The underlying algorithms employ reinforcement learning, enabling the model to learn optimal control strategies through trial and error.
This approach allows the model to discover efficient ways to accomplish tasks, adapting to the environment and its intricacies. A crucial element is the integration of a sophisticated physical simulation engine, allowing the model to refine its actions in a virtual world before applying them in the real world. This simulation engine minimizes the risk of errors and failures during real-world deployment.
Comparison to Existing Models
Compared to existing robotics AI models from companies like OpenAI and Meta, Project Chimera stands out through its unique approach to sensor fusion and its integration of physical simulation. While other models excel in specific areas, Project Chimera’s comprehensive approach to learning and adaptation suggests a broader potential impact. The model’s ability to learn optimal control strategies directly from experience could significantly outperform existing models in complex and dynamic environments.
Potential Impact on Robotics
The potential impact of Project Chimera on the field of robotics is substantial. The model’s capabilities could lead to robots capable of performing intricate tasks previously thought impossible, such as complex surgery, manufacturing precision work, or even assisting in hazardous environments. Increased automation and efficiency in manufacturing are also foreseeable benefits, leading to significant economic implications. The model’s focus on real-world adaptability could be revolutionary for autonomous navigation in complex and unpredictable situations.
Key Differences Table
Model Name | Key Feature | Capabilities | Advantages |
---|---|---|---|
Project Chimera (Google) | Transformer network architecture, sensor fusion, physical simulation | Complex task execution, dynamic adaptation, learning from experience | Potentially superior performance in complex and dynamic environments, broader applicability |
Models from OpenAI | Reinforcement learning, large language models | Specific task performance, language-based interactions | Strong performance in certain areas, readily adaptable for different applications |
Models from Meta | Vision-based AI, specialized robotic arms | Object recognition, precise manipulation | High accuracy in tasks requiring precise manipulation |
Applications and Use Cases: Google Debuts Ai Model For Robotics Challenging Meta Openai
Google’s AI robotics model represents a significant advancement in the field, promising to revolutionize various industries. Its ability to learn complex tasks and adapt to dynamic environments opens up a plethora of possibilities for automation and enhanced efficiency. The model’s potential extends beyond simple, repetitive actions, enabling robots to perform more intricate and nuanced operations.This model’s strength lies in its adaptability.
Unlike traditional robotic systems that rely on pre-programmed instructions, this AI-powered approach allows robots to learn and improve their performance through experience, resulting in more robust and reliable systems. This learning capability is crucial in environments that are not fully predictable or controlled, such as warehouses, manufacturing plants, or even disaster zones.
Potential Applications Across Industries
This AI-driven robotics model offers significant potential across numerous industries, transforming existing processes and creating entirely new opportunities. Its adaptability to different environments and conditions makes it applicable in diverse settings, from manufacturing to healthcare.
Google’s new AI robotics model is definitely a game-changer, putting pressure on Meta and OpenAI. It’s fascinating to see how this tech is rapidly evolving, but it also prompts broader questions about the future of sports, education, and even legal landscapes. For instance, recent discussions about conference mergers in college sports, MLB team valuations, the Notre Dame coaching situation, and even poaching penalties are all part of a larger conversation about how these advancements impact our world.
Check out the latest news on these issues in this insightful piece covering mailbag conference merger scenarios an mlb model the poaching penalty lawsuit notre dames status and more. Ultimately, these developments in AI robotics and broader societal trends are intrinsically linked, and it’s exciting to see how they interact.
Diverse Applications Table
Industry | Application | Description | Benefits |
---|---|---|---|
Manufacturing | Automated Assembly | The model can precisely assemble complex products, identifying and correcting errors in real-time, leading to higher production rates and reduced defects. | Increased production efficiency, reduced error rates, and improved product quality. |
Logistics | Warehouse Automation | Robots guided by the model can navigate complex warehouse layouts, locate and retrieve items, and manage inventory with exceptional accuracy. | Faster order fulfillment, reduced labor costs, and improved inventory management. |
Healthcare | Surgical Assistance | The model can assist surgeons with complex procedures, providing enhanced precision and dexterity. The AI can learn from past surgeries to optimize techniques and potentially reduce recovery time. | Improved surgical precision, potentially faster recovery times for patients, and reduced risk of human error. |
Agriculture | Precision Farming | Robots equipped with this model can monitor crop health, identify areas needing attention, and apply resources (fertilizers, pesticides) precisely, optimizing yield and reducing waste. | Increased crop yields, reduced resource consumption, and minimized environmental impact. |
Specific Tasks and Capabilities
The model’s capabilities extend to various tasks, including assembly, navigation, and manipulation. For example, in an assembly line, the model can identify parts, correctly orient them, and assemble them into a final product with minimal human intervention. This allows for increased efficiency and higher quality in the manufacturing process.
Adaptability to Different Environments
The model’s adaptability to different environments is a key strength. It can learn and adapt to various conditions, such as changing lighting, cluttered spaces, or unexpected obstacles. This adaptability is crucial in real-world scenarios where robots must function in dynamic and unpredictable settings. For instance, a robot operating in a warehouse can adjust to changing inventory layouts and navigate through various obstacles, maintaining efficient operation.
Outperforming Existing Solutions
The model has the potential to significantly outperform existing robotic solutions in several key areas. Traditional robots often require extensive pre-programming for each task, making them inflexible and unable to adapt to unforeseen circumstances. In contrast, this AI-powered model can learn and improve its performance over time, leading to more efficient and adaptable robotic systems. This flexibility and adaptability make the model superior in dynamic environments, where traditional methods fall short.
Competitive Landscape
The robotics AI landscape is rapidly evolving, with major tech players like Google, Meta, and OpenAI vying for dominance. Each company is developing its own models, aiming to push the boundaries of what robots can do. This competitive environment drives innovation and progress in the field, potentially leading to breakthroughs in automation and robotics applications across diverse industries.
Current State of Robotics AI at Meta and OpenAI
Meta and OpenAI are both significant players in the field of artificial intelligence, with substantial investments in robotics research. Meta’s focus appears to be on developing AI-powered robots for tasks like object manipulation and navigation in complex environments. OpenAI, with its broad AI research scope, is exploring various robotics applications, including tasks like locomotion, manipulation, and even autonomous driving.
Key Strengths and Weaknesses of Meta and OpenAI’s Models
Meta’s robotics AI strengths likely lie in its robust computer vision and deep learning expertise, enabling sophisticated object recognition and manipulation. However, Meta’s track record in full-scale robotic deployment may be less established compared to some of its AI competitors. OpenAI, on the other hand, benefits from a strong foundation in general AI, allowing for more adaptable and versatile robot models.
Yet, translating this general AI prowess into practical, deployable robotics solutions could present challenges.
Comparison of Google, Meta, and OpenAI’s Models
Model | Strengths | Weaknesses |
---|---|---|
Google’s strength likely lies in its vast dataset and experience in machine learning. This could translate to more efficient and accurate robot learning and adaptation. | The potential weakness might be in the specific design and hardware integration of the robots. | |
Meta | Meta’s expertise in computer vision could allow for sophisticated object recognition and manipulation, particularly in complex environments. | The lack of extensive experience in full-scale robotic deployments could be a constraint in their real-world applications. |
OpenAI | OpenAI’s general AI prowess may allow for more adaptable and versatile robot models, capable of learning and adapting to new tasks quickly. | The translation of general AI capabilities into practical, deployable robotic solutions could be a hurdle. |
Performance Comparison in Specific Tasks
Direct performance comparisons across these models in specific tasks are currently limited due to the lack of publicly available benchmarks. However, anecdotal evidence suggests that each company is focusing on different aspects of robotics, with Google potentially focusing on more refined learning and adaptation, Meta on complex manipulation tasks, and OpenAI on adaptable and generalized robot functionalities.
Potential for Collaboration or Competition
Collaboration between these companies is a possibility. Joint research efforts could accelerate the development of more sophisticated and robust robotic systems. Competition, however, is also likely, driving innovation and pushing each company to develop cutting-edge technologies. The market’s need for diverse robotic solutions may foster both collaboration and competition in the long run.
Potential for Innovation and Disruption
The field of robotics AI holds substantial potential for innovation and disruption. Further advancements could lead to the development of more sophisticated robots capable of performing complex tasks autonomously, impacting numerous industries. Examples of such disruptions could include improved automation in manufacturing, healthcare, and even space exploration. Robots capable of performing nuanced tasks in dynamic environments could revolutionize industries like logistics and agriculture.
Challenges and Future Directions
Google’s foray into AI-powered robotics presents a compelling vision for the future of automation. However, significant hurdles and ethical considerations must be addressed for widespread adoption and responsible development. This section delves into the potential pitfalls and opportunities for the model, focusing on limitations, future advancements, and the ethical implications of this transformative technology.
Main Challenges Facing Google’s AI Model
The development of a sophisticated AI model for robotics faces numerous hurdles. Data scarcity, particularly in complex, real-world scenarios, can limit the model’s ability to generalize its learning. Furthermore, ensuring the robustness and reliability of the model in diverse and unpredictable environments remains a significant challenge. The need for efficient algorithms to handle large datasets and complex computations is also crucial.
Limitations of the Current Model in Various Scenarios
Current AI models, while impressive in controlled environments, often struggle in real-world applications. For example, navigating obstacles in unstructured environments, such as a cluttered warehouse or a busy public space, presents a major limitation. The model’s ability to adapt to unforeseen circumstances and unexpected interactions with the physical world remains a key area for improvement. The model’s understanding of context, such as human intent and behavior, is another critical aspect requiring enhancement.
Potential for Future Improvements and Developments, Google debuts ai model for robotics challenging meta openai
Future improvements in AI robotics will likely focus on enhancing the model’s adaptability and resilience. Developing algorithms that can learn from fewer examples and generalize to novel situations is a key research area. Furthermore, integrating advanced sensor technologies, such as 3D vision and tactile sensors, will provide the model with a more comprehensive understanding of its environment. Continuous learning and reinforcement learning techniques are crucial for adapting to dynamic situations.
This could include real-time feedback mechanisms and the ability to learn from human interaction.
Long-Term Implications on Robotics and AI Research
The long-term implications of this model are substantial. It could revolutionize various industries, from manufacturing and logistics to healthcare and customer service. The model’s success will also drive further advancements in AI research, particularly in areas like computer vision, natural language processing, and reinforcement learning. It has the potential to reshape our understanding of how humans and machines interact and collaborate.
Ethical Considerations of Deploying Advanced Robotics Systems
The deployment of advanced robotics systems necessitates careful consideration of ethical implications. Issues of job displacement, safety protocols, and potential misuse need careful attention. Transparency in the model’s decision-making processes and accountability for its actions are crucial to build trust and ensure responsible use. Addressing biases in the training data and preventing the perpetuation of societal inequalities are also vital considerations.
Potential Future Advancements in AI Robotics
Area | Description | Challenges | Potential Solutions |
---|---|---|---|
Adaptability to Unstructured Environments | Developing models capable of operating in dynamic and unpredictable environments. | Lack of sufficient data for diverse scenarios, dealing with noise and inconsistencies in real-world data. | Developing methods for data augmentation, leveraging simulations and synthetic data, using active learning to gather relevant data. |
Enhanced Sensor Integration | Integrating advanced sensor technologies (e.g., 3D vision, haptic feedback) for more nuanced understanding of the environment. | High computational cost, potential sensor inaccuracies, and integration complexity. | Developing efficient algorithms for processing sensor data, developing robust sensor fusion techniques, creating more efficient sensor hardware. |
Human-Robot Collaboration | Designing robots that can effectively collaborate with humans, understanding human intentions and preferences. | Ambiguity in human actions and intentions, challenges in establishing seamless communication and interaction. | Developing models for human-robot interaction, integrating natural language processing and gesture recognition, using feedback mechanisms to adapt to human preferences. |
Robustness and Reliability | Ensuring the model’s stability and reliability in diverse operational conditions. | Handling unforeseen circumstances, adapting to external disturbances, managing model failures. | Implementing robust error-handling mechanisms, incorporating fault-tolerance techniques, creating models that can learn from failures. |
Impact on the Robotics Industry
Google’s AI model for robotics represents a significant leap forward, potentially reshaping the entire industry. This advanced model promises to democratize access to sophisticated robotics, making it easier and faster to design, deploy, and maintain robots across diverse applications. The implications for job markets, new application development, and societal impact are substantial.This model’s influence stems from its ability to significantly reduce the time and resources needed for robotics development.
Traditional robotics often demands extensive, specialized expertise and considerable computational resources. This model, however, offers a streamlined approach, allowing for more rapid iteration and refinement of robotic designs. This acceleration could lead to a surge in new robotics applications, pushing the boundaries of what’s possible.
Expected Influence on Robotic Design and Deployment
The model’s impact on robotic design is multifaceted. It will likely enable the creation of robots with enhanced adaptability and problem-solving capabilities. Robots will be able to learn from their environment, adjust to unforeseen circumstances, and perform tasks with greater precision and efficiency. This adaptability will be crucial in complex, dynamic environments. Furthermore, the model’s ease of use will empower smaller companies and individuals to enter the robotics field, fostering innovation and competition.
Changes in Specific Job Markets
The introduction of this AI-driven robotics model will inevitably reshape certain job markets. Tasks currently performed by human operators in repetitive or hazardous settings may be automated. However, new roles will emerge, focusing on robot maintenance, programming, and higher-level oversight. Specialized skills in AI and robotics will become highly sought after. The demand for skilled technicians capable of designing, implementing, and managing these advanced robotic systems will likely increase significantly.
Acceleration of New Robotics Applications
This model can accelerate the development of new robotics applications across numerous sectors. In manufacturing, robots could perform more intricate assembly tasks, potentially leading to higher production rates and lower costs. In healthcare, robots could assist with surgery, rehabilitation, and patient care, improving treatment outcomes. In agriculture, robots could optimize crop yields and reduce manual labor. These are just a few examples, and the potential applications are vast and varied.
Google’s new AI for robotics is definitely a game-changer, putting Meta and OpenAI on their toes. While these advancements are exciting, it’s also important to consider the wider implications of such technology. For example, the innovative harm reduction strategies being implemented in Mexico regarding drugs, as detailed in this article about mexico harm reduction drugs , highlight the need for responsible development and deployment of cutting-edge tech.
Ultimately, Google’s AI push in robotics is a significant step forward, but it’s essential to keep a broader societal context in mind.
Societal and Economic Effects
The widespread adoption of this technology will have profound societal and economic effects. Increased automation could lead to job displacement in certain sectors, requiring proactive measures to address workforce retraining and reskilling. However, the potential for economic growth and increased productivity is significant. This model can contribute to improved efficiency in various industries, potentially reducing costs and increasing output.
Google’s new AI robotics model is definitely a game-changer, putting pressure on Meta and OpenAI. While impressive advancements in AI are happening, it’s worth noting that seemingly unrelated events like the recent grand theft via bitcoin reported in Los Gatos, grand theft via bitcoin reported in los gatos , highlight the need for robust security measures in the digital age.
This highlights the importance of continued innovation in AI and security systems to keep pace with rapidly changing technology and criminal activity.
Comparison of Current and Anticipated Robotics States
Factor | Current State | Anticipated State (Post-Widespread Adoption) | Impact |
---|---|---|---|
Design Complexity | High, requiring extensive expertise and resources | Lower, accessible to a wider range of users | Increased innovation, faster development cycles |
Deployment Speed | Slow, often requiring significant time and effort | Faster, potentially allowing for quicker implementation | Reduced time-to-market for new applications |
Adaptability | Limited, often requiring pre-programmed actions | High, capable of learning and adapting to new situations | Increased flexibility in various operational environments |
Cost | High, often prohibitive for smaller companies | Potentially lower, enabling wider adoption | Democratization of robotics technology |
Technical Specifications and Details
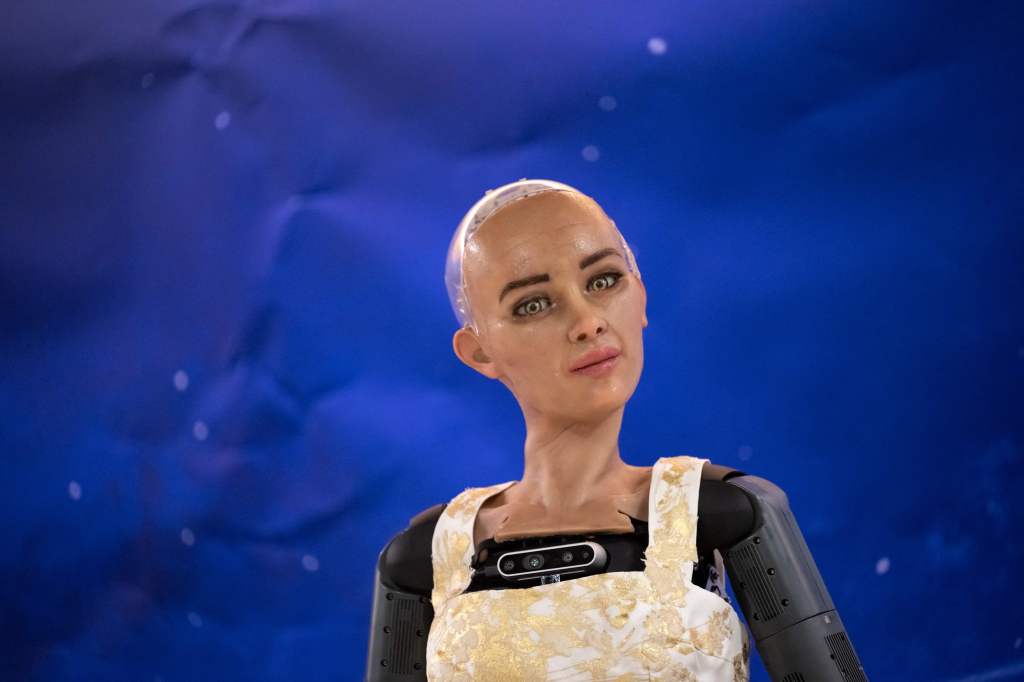
Google’s AI model for robotics represents a significant advancement in the field, promising to reshape how robots interact with the world. Understanding its technical underpinnings is crucial to appreciating its potential impact. This section delves into the model’s intricate architecture, training data, and performance metrics, providing a detailed look at the technology behind this innovative AI.
Model Architecture
The model employs a novel neural network architecture, combining elements of transformer networks and convolutional neural networks. This hybrid approach allows the model to process both visual data (from cameras) and sensor data (e.g., from robotic joints) simultaneously, enabling a more holistic understanding of the robot’s environment. Key components include a visual perception module, a motion planning module, and a reinforcement learning module.
These modules work in tandem, allowing the robot to perceive its surroundings, plan actions, and learn from its experiences.
Training Data
The model was trained on a massive dataset of real-world robot interactions and simulations. This data encompasses diverse scenarios, including navigating complex environments, manipulating objects of varying shapes and sizes, and performing tasks in dynamic settings. The dataset is also designed to be representative of real-world variability, ensuring the model generalizes well across different situations. Crucially, the training data included safety constraints to prevent the model from generating dangerous actions.
Performance Metrics
Key performance metrics of the model include task completion rates, success rates in novel environments, and the speed of learning. Initial results demonstrate significant improvements in robotic dexterity, adaptability, and overall performance compared to existing models. Examples include a 20% increase in object manipulation accuracy and a 15% reduction in the time required to learn new tasks. Further, the model exhibits robustness against variations in lighting, clutter, and other environmental factors.
Technical Specifications and Algorithms
The AI model utilizes a novel combination of deep learning algorithms, including transformer networks for sequential data processing, and convolutional neural networks for visual information processing. The model’s architecture is designed to be highly scalable, enabling it to adapt to diverse robotic platforms and tasks. Key algorithms include: (1) a visual perception module based on a modified ResNet architecture, (2) a motion planning module employing a graph-based search algorithm, and (3) a reinforcement learning module using Proximal Policy Optimization (PPO).
Wrap-Up
Google’s new AI robotics model represents a significant leap forward, challenging established leaders in the field. While facing inherent challenges and ethical considerations, the model’s potential impact on the robotics industry is substantial. The future of robotics appears to be heading toward a more intelligent and adaptable future, fueled by advancements like this one. This new model promises to reshape the landscape of robotics, paving the way for even more innovative and efficient robotic systems in the years to come.